A compilation of recent predictions indicates that artificial intelligence (AI) will likely take away as many as one billion jobs over a decade between 2023-2033. On the other hand, despite wide speculations and more than $80 billion in R&D investment, our roads and streets are yet to be full of autonomous vehicles–disrupting automobile ownership and killing jobs. Rapid early progress in developing AI solutions for automating cancer detection and prescription led to IBM’s enthusiastic investment. However, due to Premature Saturation, IBM accepted the reality upon losing USD 2 billion in building AI capability for automating cancer prescriptions. Besides, banning face recognition software by the European Parliament in public places gives chilling signals to profiting from AI possibilities. On the other hand, Elon Musk’s Full Self-Driving (FSD) technology has been suffering from class action lawsuits. Such a reality raises the issue of pervasive AI uncertainty.
AI uncertainty has been a cause of concern in policy, regulation, investment, and career decisions. Artificial intelligence (AI) refers to the human-like cognitive abilities of machines. Due to natural limitations of humans’ cognitive capability, like latency and limited scalability, AI offers excellent potential. Due to this, it’s widely speculated that AI will take over humans’ cognitive roles in using and making products to get jobs done better. Hence, there has been a high expectation for massive transformation due to the rise of AI waves– reinventing goods and services. Among many predictions, “AI will render all jobs obsolete” has fueled fear.
Despite demonstrating early progress, massive R&D investment, numerous high-profile Startups, and predictions of disruptive consequences, AI suffers from multiple uncertainties. Notable causes of AI uncertainty are technology weakness, unclear consumer preferences, misleading early progress, premature saturation, incomplete knowledge, and unpredictable operating environment.
Key Takeaways
- The underlying causes of AI uncertainty are unsettled claims of massive disruptions, misleading early progress of AI applications, and mysterious names of technology core like deep learning.
- AI uncertainty encompasses risks in job loss, premature saturation of AI technology, migration of Innovation epicenters, return on investment, winner-takes-all, privacy, and high startup mortality.
- As reality could not be fully represented through a finite set of data, a memorization-based machine learning approach through training and testing data causes AI uncertainty in a real-life environment.
- Handling artificial intelligence uncertainty has become paramount due to misleading early progress, premature saturation, mega disruptive implications possibility, and inflated expectations.
Contents
- Defining AI Uncertainty
- AI technology uncertainty
- Consumer Preference Uncertainty about AI
- AI faces crossing the chasm uncertainty
- AI disruption and job loss uncertainty
- AI investment and startups face uncertainty
- AI policy, regulation, and liability uncertainty

Defining AI Uncertainty
AI uncertainty refers to the unpredictable rise and disruptive implications of AI applications on business and jobs. Notably, in what space AI will keep rendering human capability obsolete is an uncertain concern. Besides, AI technology’s power to unleash disruptive consequences and consumer preferences towards AI applications are not evident yet. A quick demonstration of early progress and premature saturation are worsening predictions.
Uncertainty in artificial intelligence includes technology feasibility, consumer preferences, economic viability, unpredictable performance, and liability sharing.
AI technology uncertainty
There have been claims that the AI technology core is sufficiently strong to unfold mass disruptions. However, AI technology core comprising sensors, software, connectivity, computing hardware, and learning techniques like ANN alone will not unleash transformative such effects. Notably, software and learning algorithms have substantial weaknesses—making the foundation uncertain.
In reinventing existing products and processes by changing human cognitive roles with AI, Reinvention waves must cross the threshold value. Hence, AI technology must keep driving the reinvention above and beyond the existing performance level. Thus, for autonomous driving to take off and render millions of driving jobs obsolete, accident counts must fall far below the current level. Besides, the reinvention wave must show predictable performance in varying operating environments.
AI performance depends on data. Unfortunately, technology building blocks such as image, LIDAR, and acoustics sensors do not provide complete data about the environment. Besides, the representation of knowledge, in terms of the training set, is incomplete. The real-life operating environment keeps evolving, resulting in persistent deviation of the unfolding future from the past. Hence, sources of AI uncertainties include uncertain inputs, knowledge, outputs, and implications.
Due to a memorization-based learning approach through training data set, unpredictable work environment, and lack of codification of human knowledge, the AI technology base is weak. Thus, due to AI technology uncertainty, the arrival of autonomous cars, among others,has already missed several deadlines, causing investment loss and making startups bankrupt.
In many cases, reinvention waves by replacing humans’ cognitive role with AI have been prematurely saturating. Hence, the unsure rise of reinvention waves taking over humans’ cognitive functions in work has widespread implications in decision-making. Therefore, AI uncertainty encompasses risks of job loss, premature saturation, migration of innovation epicenters, return on investment, winner-takes-all, privacy, and high startup mortality.
Training set incompleteness
Machine learning weakness and complexity in mimicking human learning have been at the core of AI uncertainty. To comprehend, let’s imagine how a kid learns to recognize objects such as a horse. If we show one example of a living horse, kids can identify many variations of horses depending on color, size, health, and visibility. Upon seeing one sample, somehow, the human mind develops a scalable model that recognizes seemingly infinite variations.
Unfortunately, unlike the human mind, contemporary machine-learning techniques cannot develop scalable models from one or a few samples. Instead, it attempts to recognize objects or situations based on memorization of seemingly infinite training samples. As it’s impossible to capture a training set representing all conceivable situations, the incompleteness of the training data set is a natural limitation of contemporary AI learning algorithms.
Varying operation environment makes AI performance uncertain
Due to varying natural and man-made causes, the operating environment of AI applications like autonomous vehicles will keep varying. For example, face recognition and tracking accuracy are unpredictable due to changing outdoor lighting conditions and overlapping of faces in crowded situations. Hence, inconsistent performance of AI applications appears to be a natural outcome. However, its’ implications could be catastrophic.
For example, Tesla’s autonomous driving system got confused with the shiny white color of trucks driving ahead, leading to a crash and death. However, this is not the only incident of failure of Tesla’s fully self-driving technology (FSD). According to a Washington Post investigation, between 2019 and 2023, Tesla Autopilot was Involved in 736 Crashes, implicating 17 deaths.
Performance uncertainty and inaccuracy dent user confidence
In computational extensive decision-making exercises like taking the next move in a chase game, AI outperforms the best of the human brain with stunning accuracy, quality, and speed. However, the training data set fails to capture yet-to-be-codified or intangible human factors in real-life decision-making. Instead of cold, calculating judgment, subjective experiences, feelings, and empathy make our world a better place to live and work. Representing human qualities that emphasize empathy, ethics, and morality through training data sets appears impossible for the time being.
Besides, as explained before, unlike conventional technologies like computers, AI suffers from unpredictable performance due to changing operating environment. AI suffers from inaccuracy and unpredictability in understanding situations and taking action in most real-life cases. Hence, due to uncertain and inaccurate performance, AI applications suffer from the risk of gaining consumer confidence.
Uncertainty about premature saturation of innovation life cycle

Without facing much difficulty and spending much resources, humble effort demonstrates AI applications to mimic human-like cognitive performance in recognizing objects and making decisions. Thereby, speculation escalates about mass disruption due to the uprising of AI applications. However, as explained, due to training data set-based memorization techniques, most real-life AI applications saturate in advancement before taking over human performance. Hence, massive artificial intelligence disruption faces high uncertainty due to misleading early progress and premature saturation of AI technologies. Consequentially, artificial intelligence and the future of humans debate is non-conclusive. Besides, the prediction of job loss due to AI has been suffering from gross errors as AI technologies have been showing oscillation before crossing humans’ cognitive capabilities.
Consumer preference uncertainty about AI
How far consumers will prefer to depend entirely on machines is unclear. Will elderly people like robot nurses over their human counterparts? Based on the perception that robot nurses could be a better option for elderly care, Honda invested in Humanoid ASIMO. However, upon knowing that due to lack of warmth, older adults do not prefer robot nurses to take care of them, Honda terminated the ASIMO project. Unfortunately, Honda got this lesson upon spending over $500 million in R&D over 32 years.
Hence, it’s not surprising that robot caregivers have been failing to take off due to the lack of AI technology maturity and consumers’ tepid responses due to the absence of warmth. Due to a lack of friendliness, as older people have rejected robot nurses, there is a risk to the acceptability of human-free disease diagnosis, prescription preparation, and surgery.
Concerning autonomous vehicles, consumers would prefer to have fully autonomous ones. As they do not choose partially self-driving cars, the profitable path of gradual progression is not an option. On the other hand, there has been a high uncertainty about reaching full autonomy. Notably, full autonomy in many applications demands empathy, ethics, and morality, which are beyond the scope of the cold, calculating judgment of AI.
AI faces crossing the chasm uncertainty
In many applications, AI technology core shows quick early success. For example, even high school students demonstrate mobile robots roaming corridors without colliding with humans and other objects. Similarly, the demonstration of identifying an individual walking in the park and following in video does not take much more than simple programming work. Such demonstrations, with little effort, may even find customers serving limited purposes in a predictable environment.
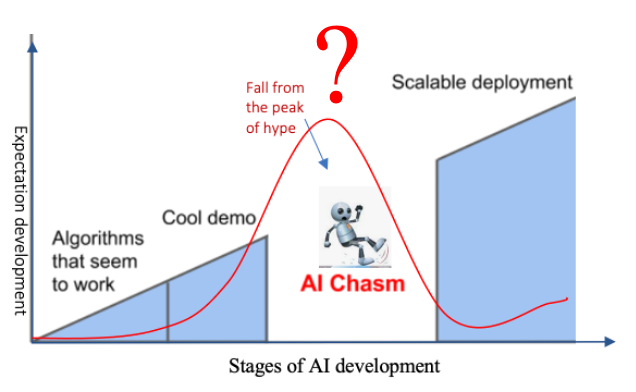
For example, students showing mobile robot demonstrations may end up getting work orders for having mobile robot platforms in a structured warehouse. Such success will likely provide the sense of penetrating more significant market segments, like making family cars autonomous, leading to accelerating investment and speculations.
However, due to premature saturation of progress, most AI innovations fail to keep growing and penetrating more significant market segments, resulting in a plunging of expectations. Hence, many highly promising AI innovations have been dying to cross the chasm, and the timeline is unpredictable due to AI technology uncertainty.
AI disruption and job loss uncertainty
Despite the demonstration of AI replacing humans, the reality is far from witnessing massive job loss due to the rise of AI-powered reinvention waves. Besides, contrary to predictions that AI will be killing mundane jobs, dead robots teach that dull jobs will likely be left to humans while automating white-collar jobs. Hence, the future of work projection with massive job loss predictions has been suffering from gross errors due to AI uncertainty.
AI investment and startups face uncertainty
Due to easy demonstrations of AI applications, there has been a surge in AI startups. Besides, there has been a trend of including machine learning techniques in various innovations. However, most of these startups have been suffering from premature saturation of their applications. Hence, due to misleading early progress, AI startups pursuing a creative wave of destruction in replacing human cognitive roles with machine learning suffer from a painful hype cycle.
AI policy, regulation, and liability uncertainty
Artificial intelligence policy framework stating decisions about the development and adoption of AI innovations in all spheres of economic, social, and public service delivery suffer from slow take off due to uncertain implications of AI. Besides high unpredictability about the rise of AI innovations and widespread fear of massive job loss, AI policy suffers from weak foundations.On the other hand, AI and privacy are growing contentious issues, resulting in the European Commission banning public deployment of face recognition software.
AI liability is an unsettled issue due to the unpredictable operating environment, making the performance of AI machines uncertain. Due to erratic behavior and the complexity of defining boundaries of responsibilities of technology developers and users, AI regulation is an unsettled issue.
There has been wide speculation that we have been at the dawn of the Fourth Industrial Revolution. Like the steam engine unleashing the first industrial revolution, AI technology is perceived as the core driving the fourth industrial revolution. However, due to the uncertain progress of AI, its transformational effect is not as visible as predicted, raising the question of when the fourth industrial revolution started. It does not necessarily mean there has been no application of human-like machine intelligence. For example, intelligent televisions take voice commands, or elevators adapt door opening with passenger movement. Such gradual migration of human-like cognitive roles to machines has been an ongoing phenomenon. However, the predictions that within a span of 10 years, massive transformation will take place, turning all jobs obsolete, suffer from the risk of being grossly incorrect due to AI uncertainty.