What does the growth of artificial intelligence (AI) related publications on arXiv by more than sixfold, from 5,478 in 2015 to 34,736 in 2020, indicate? Does it mean that memorization-based learning algorithms are heading in taking over the human race? AI publications represented 3.8% of all peer-reviewed scientific publications worldwide in 2019. It has gone up by more than three times from 1.3% in 2011. Is there a possibility that the development of artificial intelligence will reach an AI “singularity” state? At this point, machines will keep getting better by themselves. This is about AI singularity. Will humans might even be replaced by intelligent machines as the dominant “species”? This is frightening indeed. Besides, is the computing power exceeding the human brain good enough to attain superintelligence? However, despite disagreement, the possibility of scaling up the preliminary human-like intelligence to superintelligence deserves attention.
The attempt of imitating human-like intelligence in machines is neither new nor standstill. There has been steady progress in replicating human-like cognitive capabilities in real-life applications. Increasing usage of sensors in products and processes is an indication of it. But does the approach of imitating human-like intelligence using machine learning algorithms appears to be elusive to deliver the promise–like singularity? For sure, at the early stage of the technology life cycle, possibilities remain latent, creating uncertainty. Continued knowledge-gathering keeps advancing the technology. Hence, we look into the underlying science and its growth trend to predict the likely unfolding future. For sure, the trend publications and Innovation initiatives around AI are spectacular indeed. Furthermore, initiatives of leveraging this growing body of knowledge to innovate alternatives to the human role have also been exploding. But has the underlying science been advancing in supporting the maturity of AI innovations?
Promises causing provocation for reaching AI singularity:
Sensors to imitate human-like sensing capability are available at an affordable cost. Sensors like 64-megapixel cameras, thermal vision, and radar sensors have even more raw capacity than our sensing organs have. With the help of software running on tiny, powerful processors, machines can extract information from sensors data to perceive the situation and reason about actions in real-time. Furthermore, the availability of actuators allows machines to implement them without the need for the human role. Hence, building human-like machine capability is very much within our reach. Furthermore, with the available software tools, it does not take much effort to demonstrate human-like machine capability, like recognizing objects. Consequentially, it gives the impression that replacing the human role in many applications, like detecting cancer by looking into medical images, identifying crop diseases by processing images captured by smartphones, or driving automobiles is very much within reach.
In the category of human intelligence, there are three primary levels: (i) codified, (ii) tacit, and (iii) innate abilities. Developing human intelligence in machines begins with imitating codified capability, like how to solve a mathematical equation. In many aspects, we have succeeded in creating such a capability. The next level is about the imitation of experienced earned Tacit capability. Significant progress has already been made. The ultimate frontier is innate abilities. Human beings have 52 of them in four categories, including imagination and creativity.
It appears that it’s quite difficult, perhaps not impossible, to overcome some of them. But a few innate abilities like creativity and imagination seem to be out of our reach. Interestingly, human beings combine all these abilities in performing meaningful tasks. The success of automating codified capability and part of tacit ones gives the impression that AI has been heading towards imitating humans’ intelligence into machines—reaching a singularity state.
The basic concept of deep learning:

The artificial neural network is at the core of all the learning algorithms of AI. It came into existence, in 1943, with the explanation of Warren McCulloch about how neurons might work. Subsequently, in the 1950s, IBM attempted to simulate it. Simulation of the neural network consists of a set of neurons, organized as input, hidden and output layers, connected through a weight matrix. The learning takes place through the adjustment of weight. For dealing with the complexity of learning, an adequate number of hidden layers and neurons are added. Nowadays, with the help of readily available software, researchers create such a neural network by providing some design values like the number of input-output nodes and hidden layers. The availability of high-performing computing platforms, like graphics processing unit (GPU), has made the simulation of neural networks with growing hidden layers quite feasible, giving birth to deep learning.
Doing research, claiming novelty, and publishing academic articles:
Primary challenges for the researchers to imitate human intelligence are defining recognition problems in the form of feature vectors, classifying features, extracting them, and training the neural network with possible feature values. In the beginning, such AI machines learn very quickly, showing spectacular demonstration. Furthermore, researchers can cause variations in their decision of feature selection, selection of hidden layers, etc. Thus, they attempt to offer novelty to their approaches of solving a problem, say recognition of breast cancer or crop diseases through image recognition. Performance and learning behavior data could easily be collected and plotted as inspiring graphs. Besides, neural network simulation usages a set of intuitive but impressive-looking mathematical equations. Hence, it does not pose many challenges to researchers to write academic publications by claiming novelty.
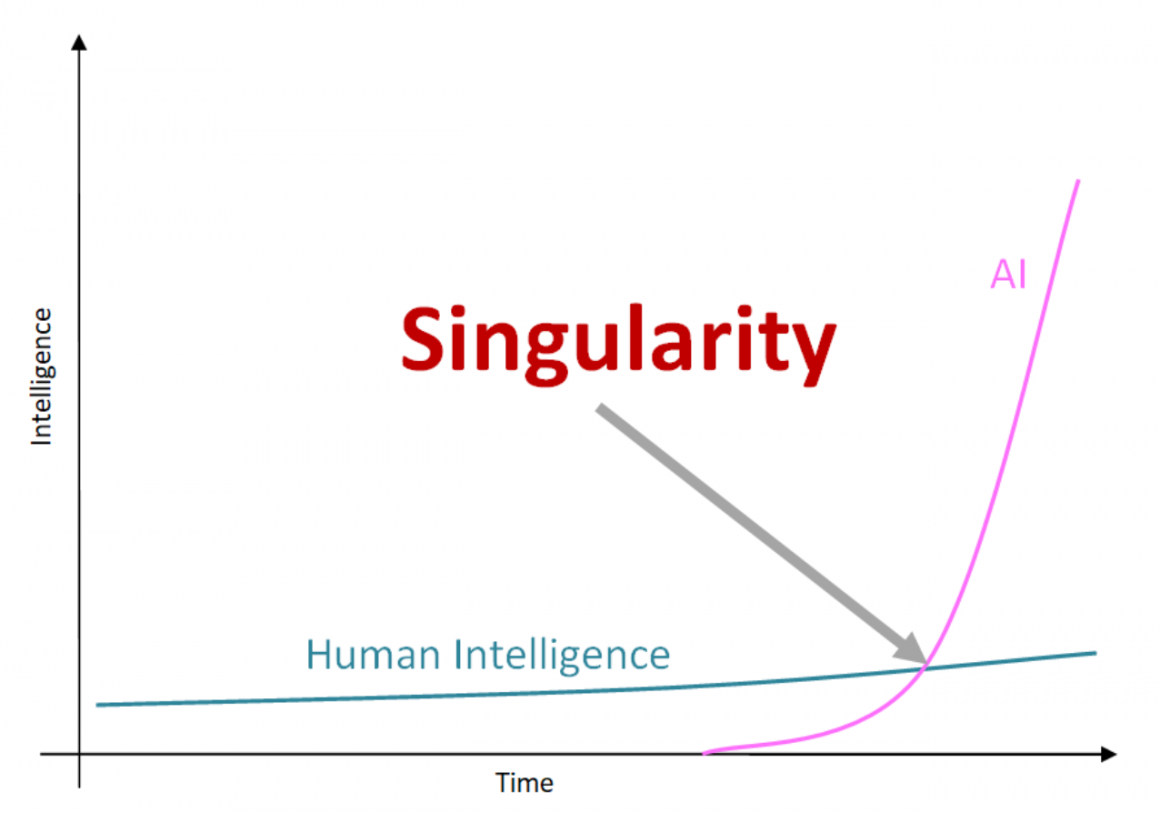
Despite showing early progress, such an approach never succeeds in imitating target human intelligence in solving real-life recognition problems. Upon attaining close to 99% accuracy, invariably, the learning machine starts oscillating, encouraging researchers to keep trying with variations of designs of feature vectors and neural net structures. Consequentially, they keep claiming novelty and publishing academic articles, taking the number to a new height. But unfortunately, the problem remains elusive, giving the option of keep increasing the number of publications. But unfortunately, the underlying science has remained unchanged for decades. Unlike the invention of electricity or other technologies, there is hardly any progress in knowledge building in basic science.
Publication counting based degrees, promotion, ranking, and fee-based publications: false indication of AI singularity
There has been an increasing acceptance of counting the number of publications for awarding degrees, giving promotions, and ranking universities. On the other hand, AI advancement for imitating human intelligence offers an easy way of making scientific publications, perhaps without making progress in solving the problem. Hence, there has been an exponential growth in the number of AI, deep learning, and machine learning publications. Besides, researchers and their organizations are willing to pay fees for publishing their novelties. Hence, there has been an exponential growth of journals as well.
Early progress triggers the startup race in AI:
To capitalize on the unfolding possibility of emerging technologies, entrepreneurs jump into the innovation race in the childhood of technology. Invariably, innovations at the early stage of the technology life cycle appear in primitive form. But along with the growth of technology, innovations also keep growing. For example, digital cameras were primitive in the 1980s. But they kept improving with the advancement of image sensor technology.
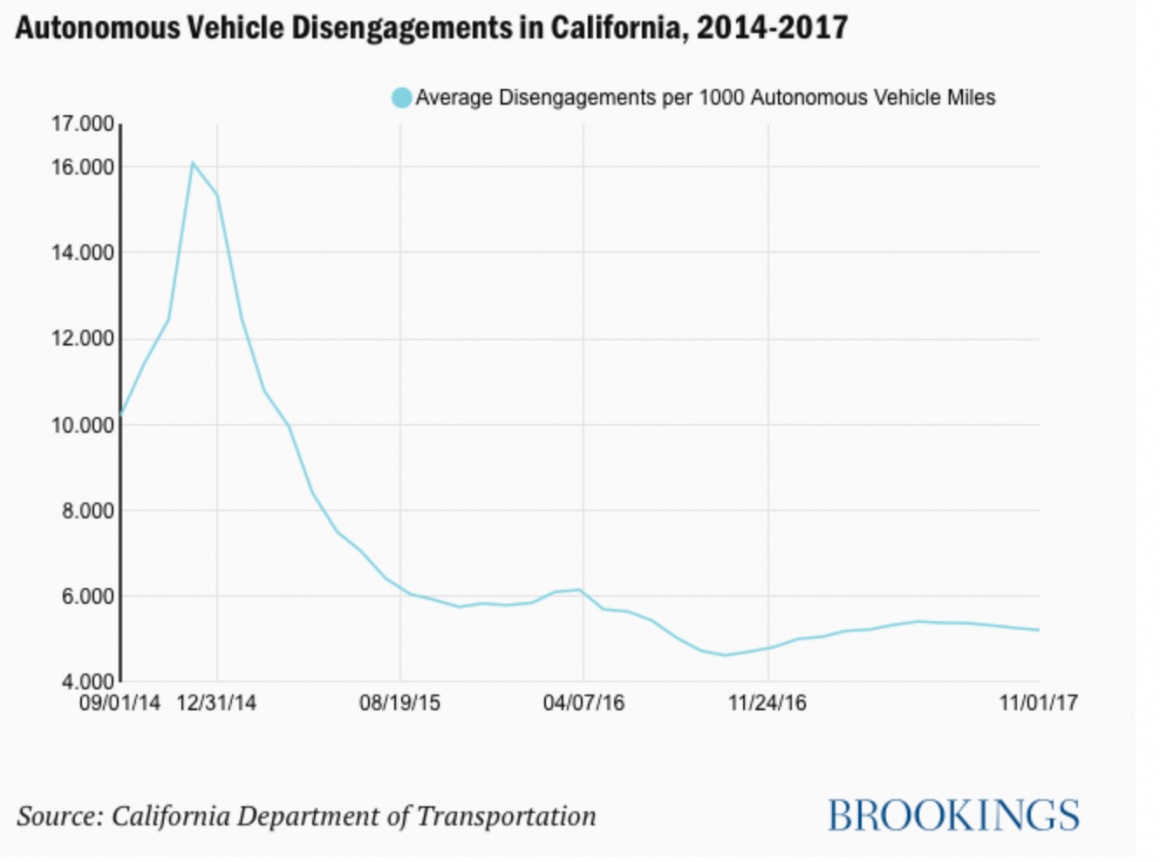
Irrespective of the greatness, every technology surfaces in a primitive form. For sure, AI is no exception. Furthermore, AI technology shows the promise of having a transformational effect. Hence, there has been a surge in innovation initiatives and Startups. But like other technology innovations, will AI innovations keep growing to surpass the threshold level of acceptance? In many applications, it’s unlikely that AI innovation will reach and surpass human intelligence. For example, after an investment of $80 billion in R&D, autonomous vehicles are yet to roll out. Oscillating behavior of the learning engine, far before overcoming the threshold level, appears to be an insurmountable barrier. With the given weak science base, it seems that many of the targeted innovations will likely fail to attain the required accuracy level.
Due to their elusive nature, many AI innovation promises run the risk of failure. Hence, this exercise of preparing data sets, designing and training learning machines, plotting performance data, claiming novelty, and making publications appear to run the risk of being a wasteful investment.
A false proposition of AI singularity: Science core must be updated
It appears that the underlying science of learning from examples as weight vector is very weak. As opposed to developing a set of equations, like other branches of science, the current machine learning approach is memorization. Furthermore, such memorization capability saturates and starts oscillating before crossing the threshold level. Hence, the likelihood of the growth memorization-based learning technique to take over humans’ ability to imagine and generate ideas appears to be impossible. Furthermore, the jubilation of AI innovations and startups appear to have a weak base to roll out and scale-up. Therefore, this current euphoria of AI out of memorization-based learning algorithms, such as deep learning, is likely heading towards dark winter.
...welcome to join us. We are on a mission to develop an enlightened community by sharing the insights of Wealth creation out of technology possibilities as reoccuring patters. If you like the article, you may encourage us by sharing it through social media to enlighten others.